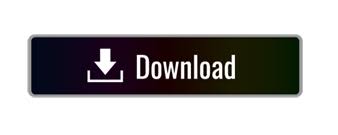
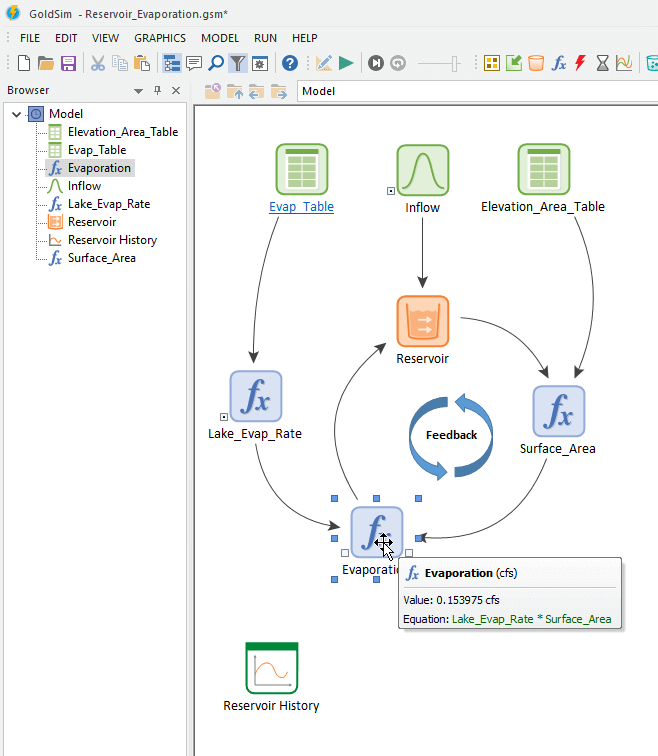
We observe the Takt Times in the same way, one as 2 days (after the story before it) and one as Zero days (after the one delivered at the same time).Īt the end of the sprint, we make note of the average Takt Time (including the Zero!). Note also the two stories delivered on the last day at the same time. The next, delivered 1 day later, therefore has a Takt Time of 1 day. The first, delivered halfway through day 2, of course has a Takt time of 1.5 days. In the example below, I’ve diagramed the delivery pattern of one sprint for a fictitious agile team.Įach story has a Takt Time, rounded to one half day. For our purposes, we want to capture this data in distinct segments, stories per week or per sprint say. The time between the completion of successive stories.


This concept becomes useful to our method when Takt Time observed as the rate at which a team completes user stories over time. You can read more about Takt Time and it’s place in the Toyota Production System here. You simply multiply the number of items required by the Takt Time. Of course it also provides an easy way to work out how long it would take to produce large batches of items. At Toyota’s Melbourne plant for example, a Takt Time of 7 minutes would mean that a new finished Camry rolls off the line every 7 minutes.īy understanding Takt Time, a manufacturer is able to run the line at a pace that is in line with demand. In production line manufacturing, Takt Time is the rate at which each finished item completes manufacture. Takt Time describes the regularity of that beat, the time in between each. Takt is a German word for a rhythm or beat. The idea uses Takt Time and mathematic Monte Carlo estimation method to determine a probable range of delivery dates.

In this post, I’m going to step through such an approach, one that I’ve been using with teams, to build a better picture of the likely delivery timeline of a medium-sized project. We always think we’ll be able to do it faster than we can and we always know that’s what people want to hear! Another wayĪn alternative to this approach is one that takes an “external” view of a team’s history and makes a forecast based on probabilistic simulation. So while it’s a really useful discussion tool, it is common to find within the resulting delivery forecasts those human traits of over optimism and a desire to please the reader. Comparative estimation done well is a wonderful facilitator of good team conversations, but it’s still an opinion, tied to the team’s analysis of the task at hand.
MONTE CARLO SIMULATION DOWNLOAD SOFTWARE
There’s great value in the discussions needed to make such an assessment, to forecast a delivery schedule for a software project.
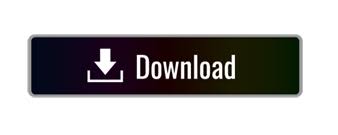